News Story
Register for ENME 737: Prognostics and Systems Health Management
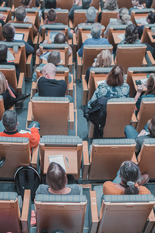
In the recent past, PHM-enabled predictive maintenance solutions were focused on complex systems (or systems of systems), such as automotive, railway transportation, and avionics, for which failure would be catastrophic. Often, those systems involved the compilation of massive amounts of historical data, the application of machine learning, and the creation of a digital “twin,” a digital rendition of the physical machine that would perform virtually like its physical counterpart. However, the ubiquity of sensors (e.g., there are 300,000 sensors in the new generations of aircraft) leads to the need for efficient processing and a relevant interpretation to gain insights from the massive volume of data. That is, synergies between the Internet of Things (IoT), data analytics, and risk assessment will ultimately determine the success of PHM.
This graduate course at the University of Maryland is one of the few in academia that covers the concepts and theory of PHM along with the latest applications. With real-life examples from academia, industry, and government, students learn and apply the latest PHM methods and tools.
CLASS TIME 9:30 AM–12:10 PM on Mondays (US Eastern) in Room 2216, J. M. Patterson Building (Bldg. 083). The class is open to graduate students, upper-level undergraduate students, and industry professionals.
AREAS OF STUDY
- The synergy between IoT, data analytics, and risk assessment
- Predictive maintenance in the IoT era
- Sensors and sensor selection for PHM
- Methods for in-situ monitoring of systems in actual life-cycle conditions
- Methods for life-cycle damage estimation
- Failure precursors and key factors for data analytics
- Machine learning methods for anomaly detection, diagnostics, and prognostics
- Big data analytics in PHM
- Uncertainty in classification and prognostics
- Methods for verification and validation of PHM capabilities
- Availability analysis and cost-benefits offered by PHM
- The role of PHM in various industry applications (consumer products, information systems, autonomous vehicles, aircraft)
Please direct all inquiries to: Professor Michael Pecht (pecht@calce.umd.edu)
Published May 23, 2018